For the uninitiated, neuromorphic computing replicates the neural structure of the human brain to create algorithms that can deal with the uncertainties of the natural world. Intel Labs has developed one of the most notable architectures in the field: the Loihi neuromorphic chip. Loihi is comprised of around 130,000 artificial neurons, which send information to each other across a “spiking” neural network (SNN). The chips had already powered a range of systems, from an smart artificial skin to an electronic “nose” that recognizes scents emitted from explosives. Intel Labs this week unveiled another application. The research unit teamed up with the Italian Institute of Technology and the Technical University of Munich to deploy Loihi in a new approach to continual learning for robotics.
Interactive learning
The method targets systems that interact with unconstrained environments, such as future robotic assistants for healthcare and manufacturing. Existing deep neural networks can struggle with object learning in these scenarios, as they require extensive well-prepared training data — and careful retraining on new objects they encounter. The new neuromorphic approach aims to overcomes these limitations. The researchers first implemented an SNN on Loihi. This architecture localizes learning to a single layer of plastic synapses. It also accounts for different views of objects by adding new neurons on demand. As a result, the learning process unfolds autonomously while interacting with the user.
Neuromorphic simulations
The team tested their approach in a simulated 3D environment. In this setup, the robot actively senses objects by moving an event-based camera that functions as its eyes. The camera’s sensor “sees” objects in a manner inspired by small fixational eye movements called “microsaccades.” If the object it views is new, the SNN representation is learned or updated. If the object is known, the network recognizes it and provides feedback to the user. The team say their method required up to 175-times lower energy to provide similar or better speed and accuracy than conventional methods running on a CPU. They now need to test their algorithm in the real-world with actual robots. “Our goal is to apply similar capabilities to future robots that work in interactive settings, enabling them to adapt to the unforeseen and work more naturally alongside humans,” Yulia Sandamirskaya, the study’s senior author, said in a statement. Their study, which was named “Best Paper” at this year’s International Conference on Neuromorphic Systems (ICONS), can be read here.
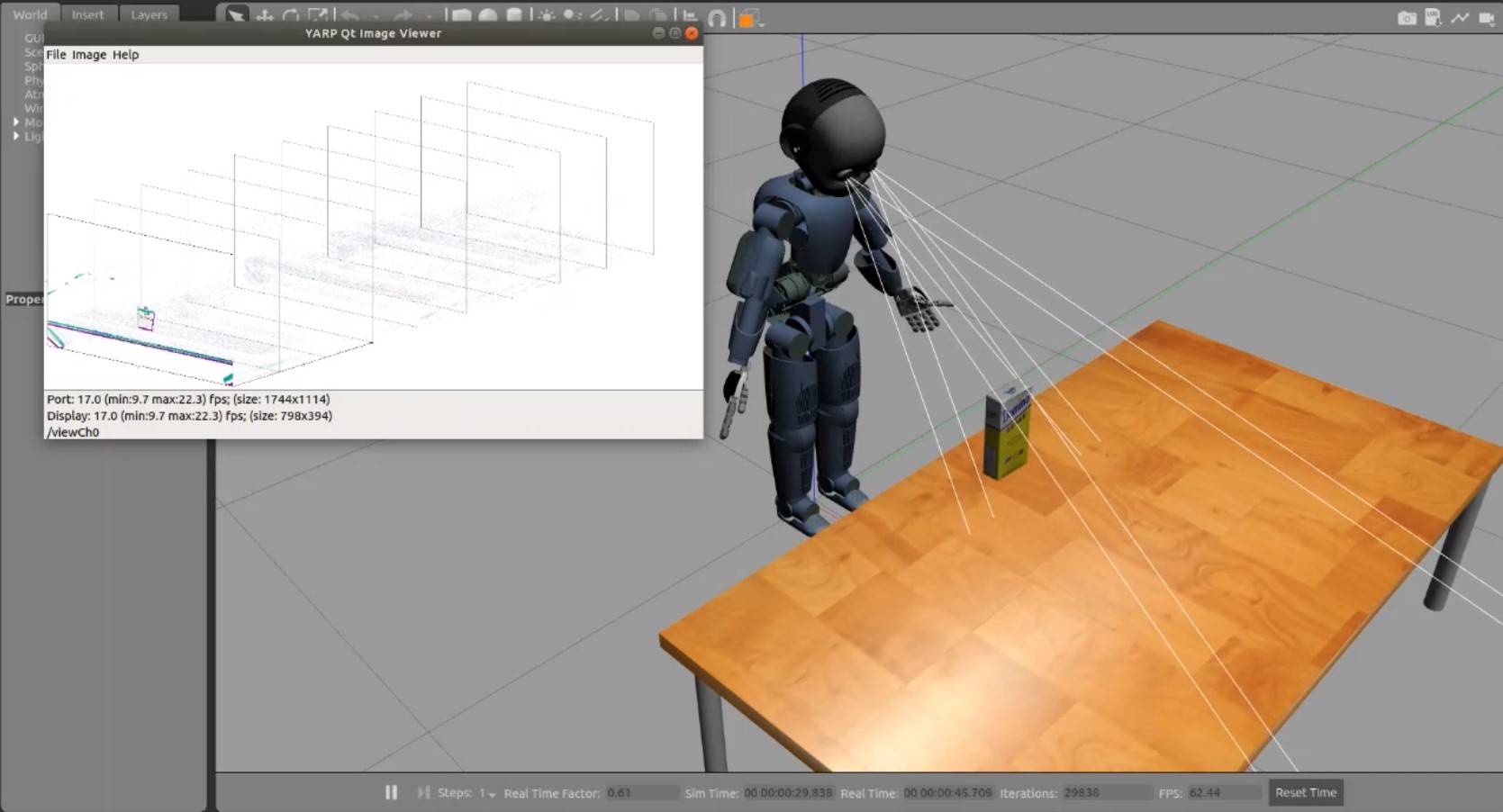